The Eberly College of Science
Department of Mathematics
Department of Mathematics
Undergraduate Programs
Acquire a foundation in pure and applied mathematics and earn a Bachelor of Arts or a Bachelor of Science degree in mathematics.
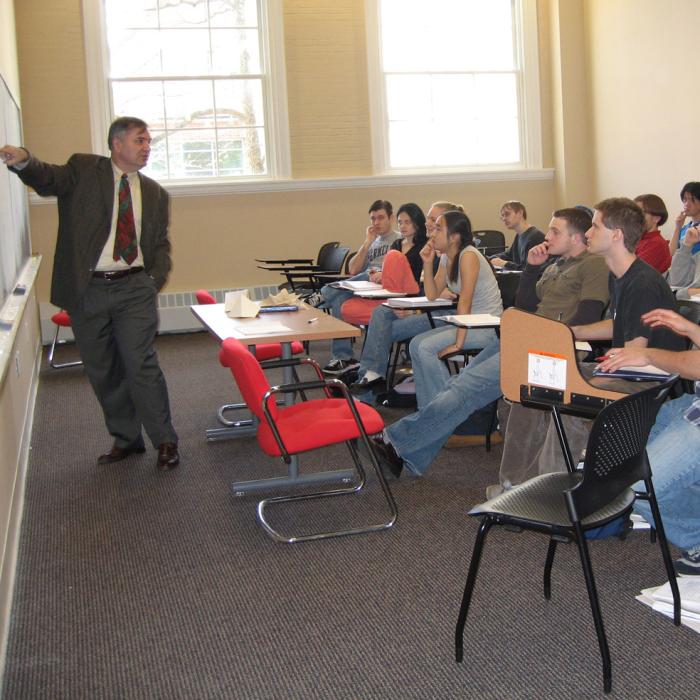
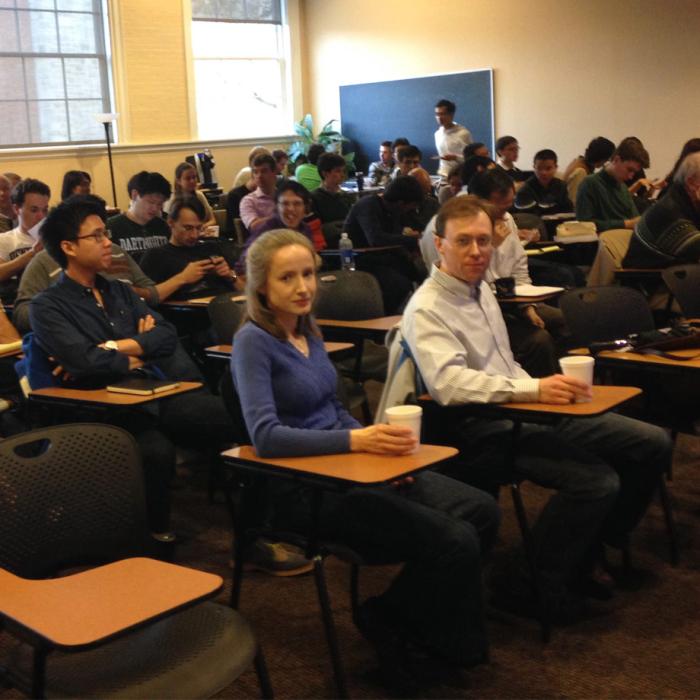
Graduate Programs
Acquire an advanced degree in mathematics and do research with some of the leading mathematicians in the world.
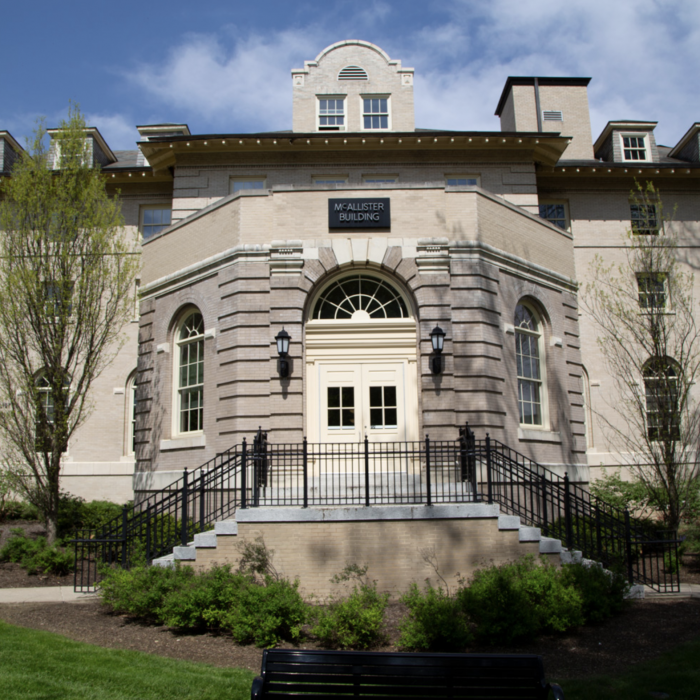